The Real Costs of Enterprise AI
By Just Admin on 11/10/2024
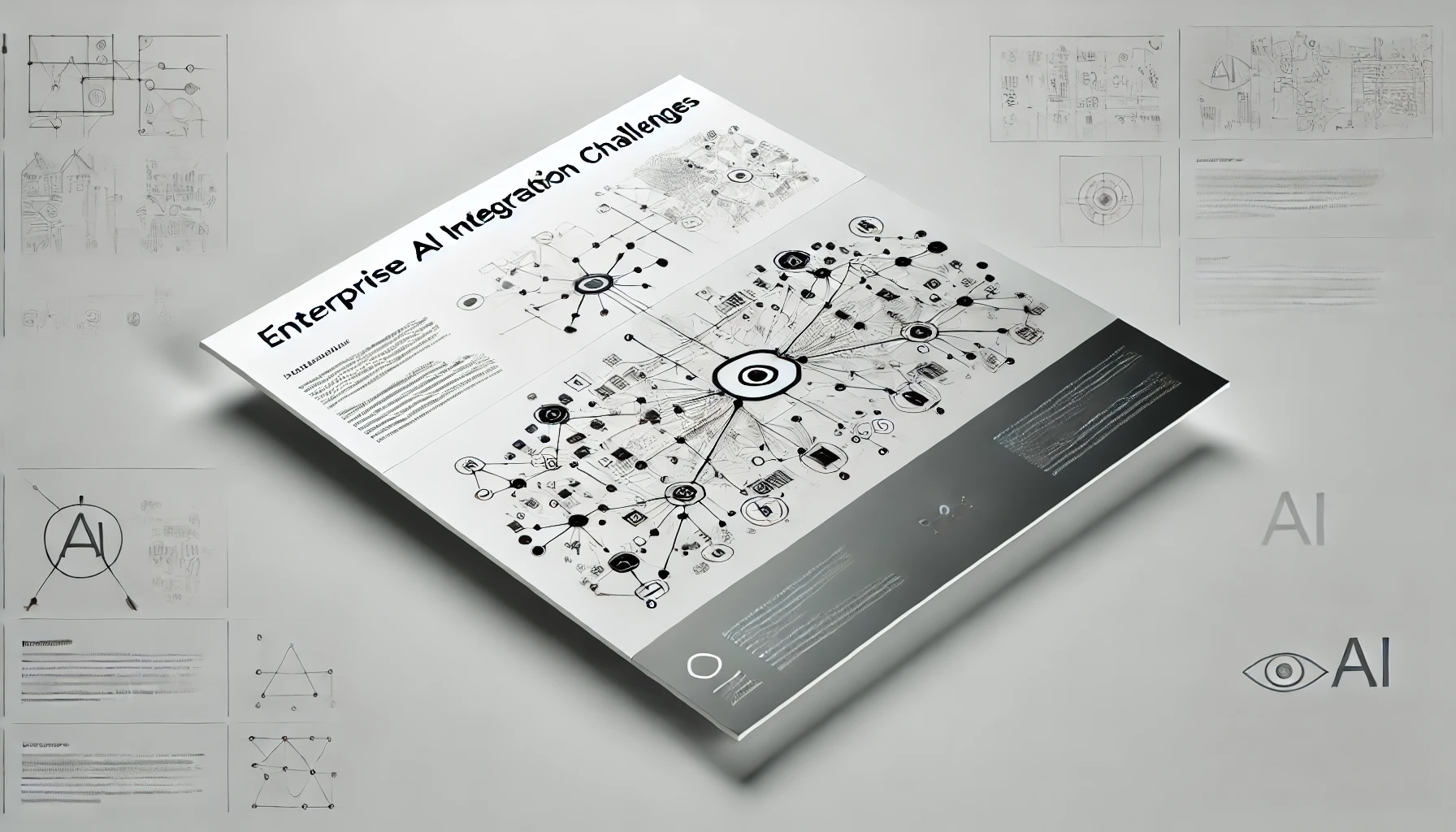
As AI becomes essential in driving enterprise efficiency and productivity, companies face a range of implementation challenges, especially when choosing between subscription-based AI solutions and API-driven custom builds. Subscription models offer quick deployment but often result in vendor lock-in, while API-based solutions provide flexibility at a high cost and with ongoing maintenance demands. Let’s dive into the hidden costs of these approaches, why they may limit enterprise scalability, and how flexible alternatives are reshaping the way enterprises approach AI.
Subscription Models: Quick Deployment, but Restrictive and Costly
Subscription-based AI models, such as Microsoft Copilot and Google Gemini for Workspace, promise rapid deployment and ease of integration with popular productivity suites like Microsoft 365 and Google Workspace. However, these solutions often come with hidden costs and significant limitations, particularly when enterprises become locked into vendor ecosystems.
-
Vendor Lock-In and Upselling Ecosystems:
It’s in the best interest of providers like Microsoft and Google to keep enterprises within their ecosystem, encouraging businesses to adopt a growing range of services to “unlock” additional features. This approach not only inflates costs over time as enterprises pay for increasingly bundled services, but it also makes it challenging and costly to switch to a competitor’s platform due to data migration complexities and workflow reconfiguration requirements. -
Cost Per User and Usage Drop-Off:
Subscription models are priced on a per-user basis, which means enterprises must calculate ROI per employee. Many organizations find that, after an initial period, the use of these tools drops significantly as generalized features fail to meet the specialized needs of different teams. This drop-off effect leads to diminished ROI, especially as the per-user fees continue accumulating without consistent, high-value usage. As companies scale, this per-user cost model can become a substantial financial burden without delivering proportional benefits.
API-Based Custom Solutions: Flexibility at the Cost of High Maintenance
For enterprises seeking customization, API-based AI solutions from providers like OpenAI, Anthropic, and Google offer greater control. However, this flexibility comes with its own set of costly challenges.
-
High Development and Maintenance Costs:
While APIs allow enterprises to customize AI tools, they require significant technical investment to implement and maintain. Creating an internal infrastructure that integrates API-based models, handling ongoing updates, and securing data within these systems demands dedicated resources and a skilled team, which can lead to high upfront and ongoing costs. -
Dependency on Single Providers and Slow Adaptation to Advancements:
In practice, enterprises often find themselves dependent on one provider when building custom API solutions. As a result, they may face similar vendor lock-in challenges as with subscription models. Even worse, integrating new features and updates from current and emerging providers is slow and labor-intensive, particularly as GenAI advancements continue to emerge almost weekly. Enterprises often end up tied to outdated versions or legacy systems because the cost of frequent updates to new models and new capabilities is prohibitive.
Infrastructure and Scalability Challenges
Enterprise AI requires more than selecting a subscription model or custom API solution. Scaling AI effectively means addressing infrastructure demands, including securing large volumes of data and managing high computational workloads. Most Custom AI solutions come with significant infrastructure costs as companies need to invest in specialized hardware, server storage, and data management processes. Additionally, security and compliance requirements drive up costs further, especially as data privacy regulations continue to evolve.
For instance, enterprises in regulated industries may require strict compliance standards, which necessitate ongoing investments in secure access protocols and data handling. These demands can add up, creating a hidden yet substantial cost layer for enterprise AI deployment.
Overcoming Vendor Lock-In and High Maintenance with a Flexible Platform
Addressing the limitations of both subscription and API-based models requires a vendor-independent, flexible platform specifically designed for enterprise use. Ideally, such a solution would allow enterprises to pay per usage directly to providers without the financial overhead of subscription fees or custom development costs. For example, with a platform like DashHub, enterprises can integrate AI from various GenAI providers—using ChatGPT for conversational workflows, Claude for data-intensive tasks, or Gemini for large document handling—all within a single, unified interface. This approach frees companies from vendor dependence and enables them to adopt the latest AI advancements seamlessly as they become available.
A flexible platform also allows enterprises to choose the right models for the right tasks without the risk of being locked into a single vendor’s ecosystem. This freedom to pivot reduces switching costs and positions organizations to remain agile as the AI landscape evolves, without the financial constraints and technical burdens that subscription and API models often impose.
Conclusion: Rethinking the Cost Structure of Enterprise AI
Enterprises looking to adopt AI effectively need to evaluate the hidden costs associated with traditional models. Subscription-based solutions may offer quick deployment, but they come with escalating costs tied to vendor lock-in. On the other hand, custom API builds provide flexibility but require ongoing investment in development and maintenance. By focusing on a vendor-independent, usage-based solution, enterprises can ensure that their AI investment remains adaptable, scalable, and aligned with their unique workflows. This strategic approach enables companies to stay competitive, innovative, and cost-efficient while maximizing the value of AI in driving internal productivity.